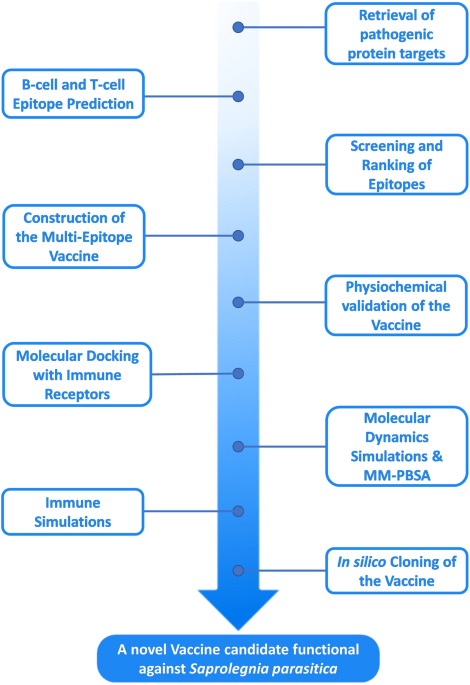
Kar, D. Chapter 1—Introduction. In Epizootic Ulcerative Fish Disease Syndrome (ed. Kar, D.) 1–19 (Academic Press, 2016).
Chong, R.S.-M. Chapter 53—Saprolegniasis. In Aquaculture Pathophysiology (eds Kibenge, F. S. B. et al.) 645–650 (Academic Press, 2022).
Pavić, D. et al. Tracing the oomycete pathogen Saprolegnia parasitica in aquaculture and the environment. Sci. Rep. 12, 16646. https://doi.org/10.1038/s41598-022-16553-0 (2022).
Lone, S. & Manohar, S. Saprolegnia parasitica, a lethal oomycete pathogen: Demands to be controlled. J. Infect. Mol. Biol. 6, 44. https://doi.org/10.17582/journal.jimb/2018/6.2.36.44 (2018).
Buchmann, K., James, B., Dalvin, S., Øvergård, A. C. & Vendramin, N. (Consejo Superior de Investigaciones Científicas (España), 2020).
Ortega, C., Fernandez, A. B., Muzquiz, J. L., Ania, S. & Gimeno, O. Health risks associated with the migration of Atlantic salmon (Salmo salar L.): An epidemiological surveillance programme in Northern Spain. Rev. Sci. Tech. 24, 887–898 (2005).
Ciepliński, M., Kasprzak, M., Grandtke, M., Giertych, M. J. & Steliga, A. Pattern of secondary infection with spp. in wild spawners of UDN-affected sea trout Salmo trutta m. (L.), the Słupia River, N Poland. Oceanol. Hydrobiol. Stud. 47, 230–238. https://doi.org/10.1515/ohs-2018-0022 (2018).
Whipps, C. M. & Kent, M. L. Chapter 41—Bacterial and fungal diseases of zebrafish. In The Zebrafish in Biomedical Research (eds Cartner, S. C. et al.) 495–508 (Academic Press, 2020).
Willoughby, L. G. & Roberts, R. J. Towards strategic use of fungicides against Saprolegnia parasitica in salmonid fish hatcheries. J. Fish Dis. 15, 1–13. https://doi.org/10.1111/j.1365-2761.1992.tb00631.x (1992).
Alderman, D. J. Malachite green: A review. J. Fish Dis. 8, 289–298. https://doi.org/10.1111/j.1365-2761.1985.tb00945.x (1985).
Srivastava, S., Sinha, R. & Roy, D. Toxicological effects of malachite green. Aquat. Toxicol. 66, 319–329. https://doi.org/10.1016/j.aquatox.2003.09.008 (2004).
Culp, S. J. et al. Carcinogenicity of malachite green chloride and leucomalachite green in B6C3F1 mice and F344 rats. Food Chem. Toxicol. 44, 1204–1212. https://doi.org/10.1016/j.fct.2006.01.016 (2006).
He, J., Mo, P., Luo, Y.-S. & Yang, P.-H. Strategies for solving the issue of malachite green residues in aquatic products: A review. Aquacult. Res. 2023, 8578570. https://doi.org/10.1155/2023/8578570 (2023).
Pipoyan, D., Stepanyan, S., Beglaryan, M., Stepanyan, S. & Mantovani, A. Health risk assessment of toxicologically relevant residues in emerging countries: A pilot study on Malachite Green residues in farmed freshwater fish of Armenia. Food Chem. Toxicol. 143, 111526. https://doi.org/10.1016/j.fct.2020.111526 (2020).
Andersson, M. G. & Cerenius, L. Pumilio homologue from Saprolegnia parasitica specifically expressed in undifferentiated spore cysts. Eukaryotic Cell 1, 105–111. https://doi.org/10.1128/ec.1.1.105-111.2002 (2002).
Matthews, E., Ellison, A. & Cable, J. Saprolegnia parasitica zoospore activity and host survival indicates isolate variation in host preference. Fungal Biol. 125, 260–268. https://doi.org/10.1016/j.funbio.2020.11.002 (2021).
Robertson, E. J. et al. Oomycete Genetics and Genomics 407–424 (Springer, 2009).
Diéguez-Uribeondo, J., Cerenius, L. & Söderhäll, K. Repeated zoospore emergence in Saprolegnia parasitica. Mycol. Res. 98, 810–815. https://doi.org/10.1016/S0953-7562(09)81060-5 (1994).
Wawra, S. et al. Host-targeting protein 1 (SpHtp1) from the oomycete Saprolegnia parasitica translocates specifically into fish cells in a tyrosine-O-sulphate-dependent manner. Proc. Natl. Acad. Sci. 109, 2096–2101. https://doi.org/10.1073/pnas.1113775109 (2012).
Trusch, F. et al. Cell entry of a host-targeting protein of oomycetes requires gp96. Nat. Commun. 9, 2347. https://doi.org/10.1038/s41467-018-04796-3 (2018).
Rezinciuc, S., Sandoval-Sierra, J. V., Ruiz-León, Y., van West, P. & Diéguez-Uribeondo, J. Specialized attachment structure of the fish pathogenic oomycete Saprolegnia parasitica. PLoS ONE 13, e0190361. https://doi.org/10.1371/journal.pone.0190361 (2018).
Srivastava, V., Rezinciuc, S. & Bulone, V. Quantitative proteomic analysis of four developmental stages of Saprolegnia parasitica. Front. Microbiol. 8, 2658. https://doi.org/10.3389/fmicb.2017.02658 (2017).
Kumar, S., Mandal, R. S., Bulone, V. & Srivastava, V. Identification of growth inhibitors of the fish pathogen Saprolegnia parasitica using in silico subtractive proteomics, computational modeling, and biochemical validation. Front. Microbiol. 11, 571093. https://doi.org/10.3389/fmicb.2020.571093 (2020).
Kuang, G., Bulone, V. & Tu, Y. Computational studies of the binding profile of phosphoinositide PtdIns (3,4,5) P3 with the pleckstrin homology domain of an oomycete cellulose synthase. Sci. Rep. 6, 20555. https://doi.org/10.1038/srep20555 (2016).
Saldanha, L., Langel, Ü. & Vale, N. In silico studies to support vaccine development. Pharmaceutics 15, 654 (2023).
Vita, R. et al. The Immune Epitope Database (IEDB): 2018 update. Nucleic Acids Res. 47, D339–D343. https://doi.org/10.1093/nar/gky1006 (2019).
Andreatta, M. & Nielsen, M. Gapped sequence alignment using artificial neural networks: Application to the MHC class I system. Bioinformatics 32, 511–517. https://doi.org/10.1093/bioinformatics/btv639 (2016).
Nielsen, M. & Lund, O. NN-align. An artificial neural network-based alignment algorithm for MHC class II peptide binding prediction. BMC Bioinform. 10, 296. https://doi.org/10.1186/1471-2105-10-296 (2009).
Doytchinova, I. A. & Flower, D. R. VaxiJen: A server for prediction of protective antigens, tumour antigens and subunit vaccines. BMC Bioinform. 8, 4. https://doi.org/10.1186/1471-2105-8-4 (2007).
Doytchinova, I. & Flower, D. Bioinformatic approach for identifying parasite and fungal candidate subunit vaccines. Open Vaccine J. 1, 22–26. https://doi.org/10.2174/1875035400801010022 (2008).
Shey, R. A. et al. In-silico design of a multi-epitope vaccine candidate against onchocerciasis and related filarial diseases. Sci. Rep. 9, 4409. https://doi.org/10.1038/s41598-019-40833-x (2019).
Chen, X., Zaro, J. L. & Shen, W.-C. Fusion protein linkers: Property, design and functionality. Adv. Drug Deliv. Rev. 65, 1357–1369. https://doi.org/10.1016/j.addr.2012.09.039 (2013).
Mittal, A., Sasidharan, S., Raj, S., Balaji, S. N. & Saudagar, P. Exploring the zika genome to design a potential multiepitope vaccine using an immunoinformatics approach. Int. J. Peptide Res. Therap. 26, 2231–2240. https://doi.org/10.1007/s10989-020-10020-y (2020).
Yano, A. et al. An ingenious design for peptide vaccines. Vaccine 23, 2322–2326. https://doi.org/10.1016/j.vaccine.2005.01.031 (2005).
Gu, Y. et al. Vaccination with a paramyosin-based multi-epitope vaccine elicits significant protective immunity against Trichinella spiralis infection in mice. Front. Microbiol. 8, 475. https://doi.org/10.3389/fmicb.2017.01475 (2017).
Yang, Y. et al. In silico design of a DNA-based HIV-1 multi-epitope vaccine for Chinese populations. Hum. Vaccines Immunotherap. 11, 795–805. https://doi.org/10.1080/21645515.2015.1012017 (2015).
Sarkar, B., Ullah, M. A., Johora, F. T., Taniya, M. A. & Araf, Y. Immunoinformatics-guided designing of epitope-based subunit vaccines against the SARS coronavirus-2 (SARS-CoV-2). Immunobiology 225, 151955. https://doi.org/10.1016/j.imbio.2020.151955 (2020).
Livingston, B. et al. A rational strategy to design multiepitope immunogens based on multiple Th lymphocyte epitopes1. J. Immunol. 168, 5499–5506. https://doi.org/10.4049/jimmunol.168.11.5499 (2002).
Mansoor, S., Baek, M., Juergens, D., Watson, J. L. & Baker, D. Zero-shot mutation effect prediction on protein stability and function using RoseTTAFold. Protein Sci. 32, e4780. https://doi.org/10.1002/pro.4780 (2023).
Lahiri, T., Singh, K., Pal, M. K. & Verma, G. Protein structure validation using a semi-empirical method. Bioinformation 8(20), 984–987. https://doi.org/10.6026/97320630008984 (2012).
Kiefer, F., Arnold, K., Künzli, M., Bordoli, L. & Schwede, T. The SWISS-MODEL repository and associated resources. Nucleic Acids Res. 37, D387–D392. https://doi.org/10.1093/nar/gkn750 (2009).
Wiederstein, M. & Sippl, M. J. ProSA-web: Interactive web service for the recognition of errors in three-dimensional structures of proteins. Nucleic Acids Res. 35, W407–W410. https://doi.org/10.1093/nar/gkm290 (2007).
Garg, V. K. et al. MFPPI—Multi FASTA ProtParam interface. Bioinformation 12(2), 74–77. https://doi.org/10.6026/97320630012074 (2016).
Dimitrov, I., Bangov, I., Flower, D. R. & Doytchinova, I. AllerTOP v.2—A server for in silico prediction of allergens. J. Mol. Model. 20, 2278. https://doi.org/10.1007/s00894-014-2278-5 (2014).
Garg, A. & Gupta, D. VirulentPred: A SVM based prediction method for virulent proteins in bacterial pathogens. BMC Bioinform. 9, 62. https://doi.org/10.1186/1471-2105-9-62 (2008).
Hebditch, M., Carballo-Amador, M. A., Charonis, S., Curtis, R. & Warwicker, J. Protein–Sol: A web tool for predicting protein solubility from sequence. Bioinformatics 33, 3098–3100. https://doi.org/10.1093/bioinformatics/btx345 (2017).
Ponomarenko, J. et al. ElliPro: A new structure-based tool for the prediction of antibody epitopes. BMC Bioinform. 9, 514. https://doi.org/10.1186/1471-2105-9-514 (2008).
Rødland, E. K., Ager-Wick, E., Halvorsen, B., Müller, F. & Frøland, S. S. Toll like receptor 5 (TLR5) may be involved in the immunological response to Aspergillus fumigatus in vitro. Med. Mycol. 49, 375–379. https://doi.org/10.3109/13693786.2010.531772 (2011).
Akhtar, N., Joshi, A., Kaushik, V., Kumar, M. & Mannan, M.A.-U. In-silico design of a multivalent epitope-based vaccine against Candida auris. Microbial Pathog. 155, 104879. https://doi.org/10.1016/j.micpath.2021.104879 (2021).
Akhtar, N., Singh, A., Upadhyay, A. K. & Mannan, M.A.-U. Design of a multi-epitope vaccine against the pathogenic fungi Candida tropicalis using an in silico approach. J. Genet. Eng. Biotechnol. 20, 140. https://doi.org/10.1186/s43141-022-00415-3 (2022).
Kozakov, D. et al. The ClusPro web server for protein–protein docking. Nat. Protoc. 12, 255–278. https://doi.org/10.1038/nprot.2016.169 (2017).
Yuan, S., Chan, H. C. S. & Hu, Z. Using PyMOL as a platform for computational drug design. WIREs Comput. Mol. Sci. 7, e1298. https://doi.org/10.1002/wcms.1298 (2017).
Abraham, M. J. et al. GROMACS: High performance molecular simulations through multi-level parallelism from laptops to supercomputers. SoftwareX 1, 19–25. https://doi.org/10.1016/j.softx.2015.06.001 (2015).
Van Der Spoel, D. et al. GROMACS: Fast, flexible, and free. J. Comput. Chem. 26, 1701–1718. https://doi.org/10.1002/jcc.20291 (2005).
Witeska, M., Kondera, E., Ługowska, K. & Bojarski, B. Hematological methods in fish—Not only for beginners. Aquaculture 547, 737498. https://doi.org/10.1016/j.aquaculture.2021.737498 (2022).
Olson, K. R. & Hoagland, T. M. Effects of freshwater and saltwater adaptation and dietary salt on fluid compartments, blood pressure, and venous capacitance in trout. Am. J. Physiol. Regul. Integr. Compar. Physiol. 294, R1061–R1067. https://doi.org/10.1152/ajpregu.00698.2007 (2008).
Vasylkiv, O. Y., Kubrak, O. I., Storey, K. B. & Lushchak, V. I. Catalase activity as a potential vital biomarker of fish intoxication by the herbicide aminotriazole. Pesticide Biochem. Physiol. 101, 1–5. https://doi.org/10.1016/j.pestbp.2011.05.005 (2011).
Yasui, G. S. et al. Flow cytometric analysis from fish samples stored at low, ultra-low and cryogenic temperatures. Cryobiology 95, 68–71. https://doi.org/10.1016/j.cryobiol.2020.06.004 (2020).
Valdés-Tresanco, M. S., Valdés-Tresanco, M. E., Valiente, P. A. & Moreno, E. gmx_MMPBSA: A new tool to perform end-state free energy calculations with GROMACS. J. Chem. Theory Comput. 17, 6281–6291. https://doi.org/10.1021/acs.jctc.1c00645 (2021).
Rapin, N., Lund, O. & Castiglione, F. Immune system simulation online. Bioinformatics 27, 2013–2014. https://doi.org/10.1093/bioinformatics/btr335 (2011).
Grote, A. et al. JCat: A novel tool to adapt codon usage of a target gene to its potential expression host. Nucleic Acids Res. 33, W526–W531. https://doi.org/10.1093/nar/gki376 (2005).
Choi, S.-Y., Ro, H. & Yi, H. A prerequisite for cloning. In DNA Cloning: A Hands-On Approach (eds Choi, S.-Y. et al.) 5–28 (Springer, 2019).
Pizza, M. et al. Identification of vaccine candidates against serogroup B meningococcus by whole-genome sequencing. Science 287, 1816–1820. https://doi.org/10.1126/science.287.5459.1816 (2000).
Sette, A. & Rappuoli, R. Reverse vaccinology: Developing vaccines in the era of genomics. Immunity 33, 530–541. https://doi.org/10.1016/j.immuni.2010.09.017 (2010).
Srivastava, P. & Jain, K. C. Computer aided reverse vaccinology: A game-changer approach for vaccine development. Comb. Chem. High Through. Screen. 26, 1813–1821. https://doi.org/10.2174/1386207325666220930124013 (2023).
- SEO Powered Content & PR Distribution. Get Amplified Today.
- PlatoData.Network Vertical Generative Ai. Empower Yourself. Access Here.
- PlatoAiStream. Web3 Intelligence. Knowledge Amplified. Access Here.
- PlatoESG. Carbon, CleanTech, Energy, Environment, Solar, Waste Management. Access Here.
- PlatoHealth. Biotech and Clinical Trials Intelligence. Access Here.
- Source: https://www.nature.com/articles/s41598-024-52223-z